Exploring the Different Sales Pipeline Forecast Models
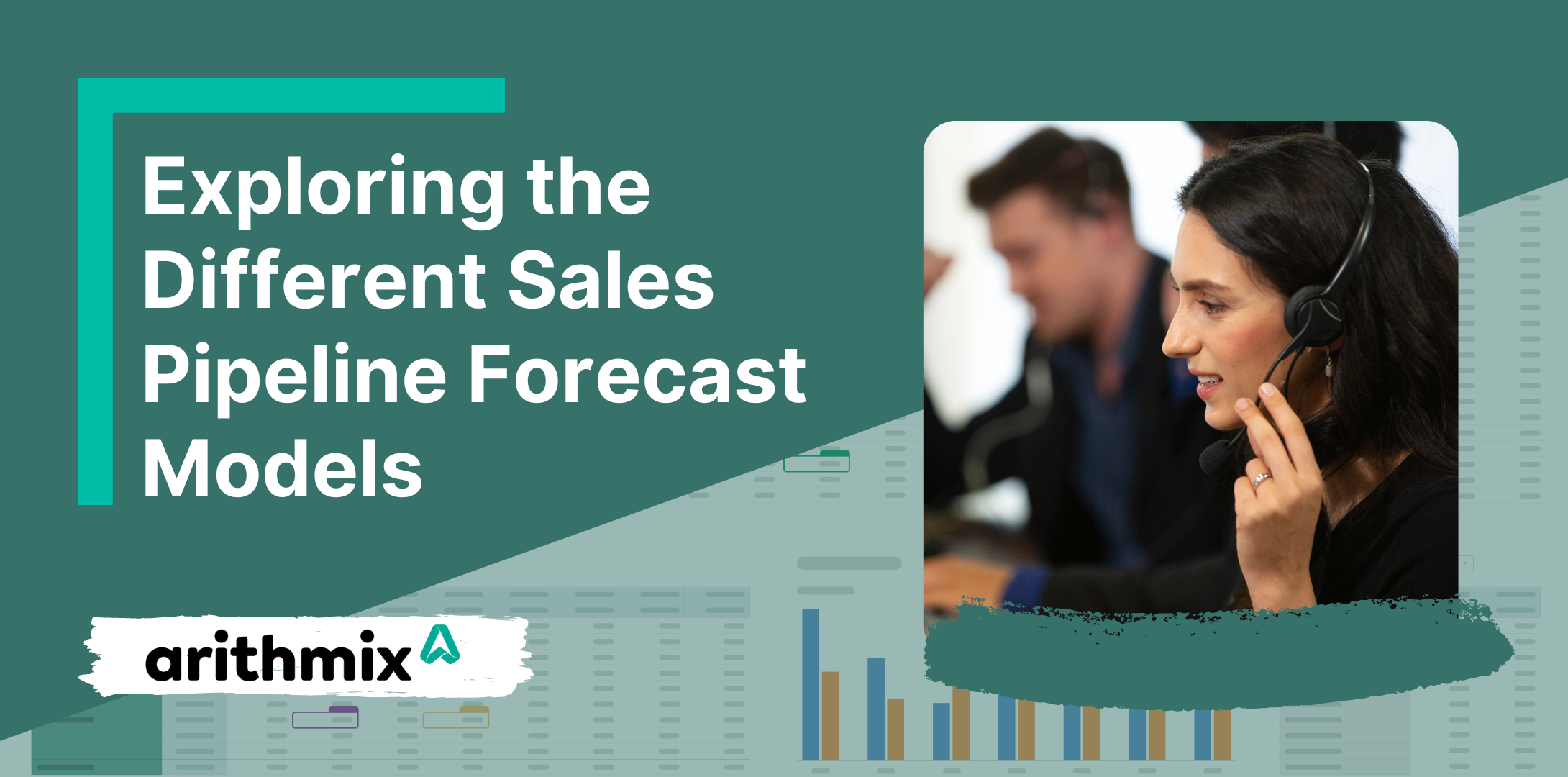
Sales pipeline forecasting is a powerful tool for businesses of all sizes. By accurately predicting sales and marketing strategies, you can make more informed decisions to maximize sales and profits. But how do you choose the right model?
By understanding the different sales pipeline models, you'll be able to optimize your strategies to effectively achieve your desired outcomes.
In this article, we will explore the various types of sales pipeline forecasting models, their benefits, common techniques used to analyze data, common strategies, and how to leverage technology to improve accuracy. Additionally, we will discuss some of the challenges associated with sales pipeline forecasting and best practices for implementing a successful model. By understanding all of these components and utilizing the proper tools (such as Arithmix's Sales Pipeline Forecast Template), businesses are sure to increase their overall success and profitability through successful sales pipeline forecasting.
Introduction to Sales Pipeline Forecasting
Sales pipeline forecasting is the process of predicting future sales pipeline performance using historical data. It involves assessing customer trends, such as purchase patterns, and predicting their potential purchases in the future. By leveraging data-driven analyses and precise algorithms, companies can project their future sales and optimally plan their strategies, such as when to reach out to existing customers to order again or renew their contract. This form of forecasting provides companies with valuable insights into their customer base, allowing them to better understand customer behavior and maximize their marketing efforts.
Sales pipeline forecasting can also be used to identify potential opportunities for growth. By analyzing customer data, companies can identify areas where they can increase their sales and target new customers. Additionally, sales pipeline forecasting can help companies identify areas where they can improve their customer service and increase customer satisfaction. By understanding customer needs and preferences, companies can better tailor their products and services to meet customer demands.
Benefits of Sales Pipeline Forecasting
The primary benefit of sales pipeline forecasting is that it helps businesses accurately forecast customer needs ahead of time. This allows them to effectively plan and tailor their strategies (such as when to hire or when to enter a new territory) to meet those needs and reach their sales targets.
It also provides companies with actionable insights into customer behaviors, which can be used to create more effective marketing campaigns. This can further increase their sales by targeting the most likely customer segments in an effective manner.
Sales pipeline forecasting also helps businesses identify potential opportunities for growth. By analyzing customer data, businesses can identify areas where they can expand their product offerings or services. This can help them capitalize on new markets and increase their overall sales.
Additionally, sales pipeline forecasting can help businesses identify areas where they can improve their customer service, which can lead to increased customer satisfaction and loyalty.
Types of Sales Pipeline Models
There are several types of sales pipeline models, generally falling into four main categories--each with its own advantages and disadvantages.
1. Qualitative models
Qualitative models rely on subjective input, such as expert opinions, industry trends, the sales team's experiences, and customer feedback, to predict sales performance. These models are often used when historical data is limited or unreliable--such as when entering a new market or launching a brand new product--or when the market is undergoing significant changes.
Qualitative forecasting models are typically less precise than quantitative models, but they can provide valuable insights into customer behavior, emerging market trends, and other factors that affect sales performance. Some common techniques used in qualitative forecasting include the Delphi method, which involves collecting input from a group of experts, and the sales force composite method, which involves gathering input from the sales team to create a consensus forecast.
2. Quantitative models
Quantitative models are more data-driven, leveraging patterns in financials and industry trends in order to form conclusions and forecast future sales. These models analyze past sales data and trends to predict future outcomes. They take into account various factors such as market demand, customer behavior, and economic indicators to generate a forecast.
One common quantitative forecasting model is time series analysis, which uses historical sales data to identify patterns and trends over time. Another approach is regression analysis, which examines the relationship between sales and various factors such as marketing spend, pricing, and customer demographics. These models are often used in conjunction with qualitative models to provide a more complete and accurate sales forecast.
3. Hybrid models
Hybrid models combine elements of both qualitative and quantitative models, allowing for a more comprehensive understanding of customer trends. These models rely on quantitative data analysis, such as historical sales data, lead conversion rates, and average deal size, to make initial projections. Then, qualitative insights from sales representatives, marketing teams, and industry experts are used to refine the projections and adjust for any external factors that may impact sales. This approach allows for a more nuanced and comprehensive understanding of the sales pipeline and helps to account for variables that are difficult to quantify.
Hybrid models can be especially useful in industries with rapidly changing markets or where external factors have a significant impact on sales, as they can adapt to new information and adjust predictions accordingly. These models are often used in larger organizations, as they require more resources and data to be effective.
4. Predictive models
Predictive models use machine learning algorithms to predict customer behavior and identify potential opportunities. For example, predictive models can analyze a vast amount of data from various sources, such as social media and website traffic, to identify patterns and make predictions based on those patterns. These models are becoming increasingly popular, especially for businesses with complex processes or large amounts of data, as they can provide valuable insights into customer behavior.
Analyzing Data with Sales Pipeline Forecasting Models
Once the data is collected and analyzed, the next step is to develop an accurate sales pipeline forecasting model. This process involves assessing the data sets and the correlations between customer behavior and other metrics. Machine learning algorithms can be used to accurately assess the data sets and create predictive models that are accurate and reliable. Businesses can then use these models to accurately predict customer behavior in the near and long-term future.
The accuracy of the sales pipeline forecasting model is dependent on the quality of the data collected. It is important to ensure that the data is up-to-date and relevant to the customer's current situation. Additionally, the data should be collected from multiple sources to ensure accuracy. By collecting data from multiple sources, businesses can gain a better understanding of customer behavior and create more accurate models.
Understanding the Impact of Different Sales Strategies on Pipeline Forecasting
Undergoing a comprehensive sales process analysis is imperative for any company to determine the most effective strategy suited for their specific business objectives. Factors such as customer segmentation, budget constraints, market conditions and competitive pressures should all be taken into consideration when developing a successful sales strategy. By understanding how different strategies impact sales pipeline forecasting, companies can determine which strategies they should focus on in order to achieve their desired outcomes.
For example, a company may choose to focus on a customer-centric approach, which involves understanding the needs of the customer and tailoring the sales process to meet those needs. This strategy can help to increase customer loyalty and satisfaction, resulting in higher sales and a more accurate pipeline forecast. Alternatively, a company may choose to focus on a more aggressive sales approach, which involves pushing for a sale as quickly as possible. While this strategy may result in a higher number of sales in the short-term, it may not be as effective in the long-term, as customers may not be as satisfied with the product or service.
Leveraging Technology to Improve Pipeline Accuracy and Efficiency
Technology has revolutionized the way companies approach sales pipeline forecasting. By utilizing software programs, such as Arithmix's free Sales Pipeline Forecast Template and other automated systems, companies can accurately collect and store data at unprecedented speeds and with greater accuracy. Automated systems can also be employed to identify patterns in data sets, perform advanced analytics, and develop accurate predictive models. All of these automated processes help save time and money, while improving accuracy.
Best Practices for Implementing a Successful Sales Pipeline Forecasting Model
Businesses must ensure that their sales pipeline forecasting models are accurate and reliable in order for them to see success in their sales objectives. To ensure accuracy and reliability, companies should follow best practice guidelines. They should define a consistent methodology for collecting data and implementing predictive models, track changes in customer behavior regularly, and keep meticulous records of all data collected. Additionally, they must set realistic goals in their model evaluation process in order to adequately assess its performance.
Challenges with Sales Pipeline Forecasting and How to Overcome Them
Despite its numerous benefits, there are several challenges associated with sales pipeline forecasting that must be addressed in order for businesses to stay competitive. One common challenge is predicting customer needs accurately due to constantly changing trends. Companies must continually update and refine their predictive models in order to respond quickly to changing trends in customer behavior. Additionally, companies must address such challenges as incomplete data sets or data inaccuracies that can lead to unreliable predictions. By understanding these challenges and implementing the necessary measures to address them, businesses can ensure that their sales pipeline forecasting models are reliable and accurate.
Conclusion
Sales pipeline forecasting is an important tool for businesses of all sizes. By accurately predicting customer behavior and tailoring their strategies accordingly, companies can reap significant rewards. By understanding the different types of models available, using effective data-driven techniques, leveraging technology, and following best practices, businesses can implement successful models that are both accurate and reliable. Furthermore, by understanding some of the challenges associated with sales pipeline forecasting, companies can mitigate risk associated with inaccurate predictions.
You can use Arithmix's Sales Pipeline Forecast Template, free, today.